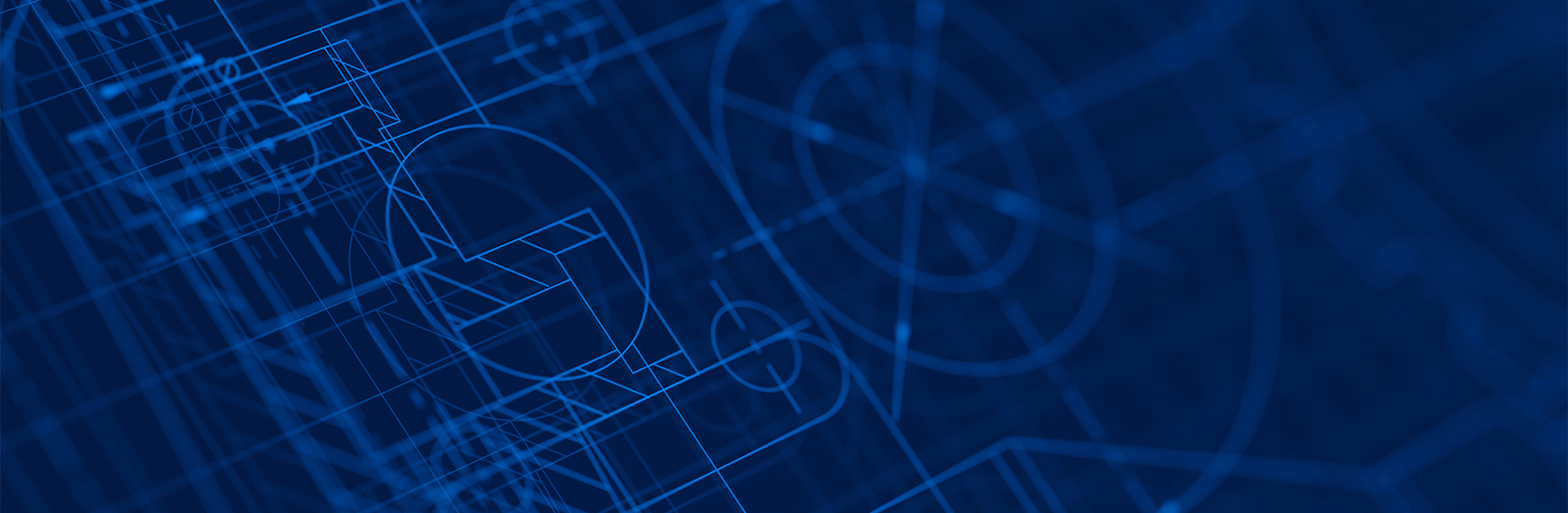
Volume modelling
Machine learning-powered modelling of production shape and volume provides improved certainty when compared to industry-standard linear regression.
Offtake and hedge optimization
Hourly settlement presents a considerable financial risk to owners when factors impacting production shape and volume, such as the variability in intra-day energy production, are not properly considered.
By using modelled hourly resource to review each month-hour pair, Clir provides valuable insight into the suitability of hedge values. Layering market intelligence on loss factor variability across the industry enables values to be further tuned for technology and asset location.
This analysis enables owners to assess the likelihood of a project failing to meet the hedged quantity at any given hour. It also offers the ability for owners to simulate the hedge settlement for any chosen value.
Business interruption
The volume model can be used to calculate the uncertainty in expected generation over different return periods for business interruption insurance. This also supports the sizing of business interruption risk exposure to ensure coverage and risk management are optimal.
Estimate lost energy during outages
The probabilistic hourly resource distribution can be used to optimize outage planning and estimate lost energy during planned or unplanned farm-wide outages.
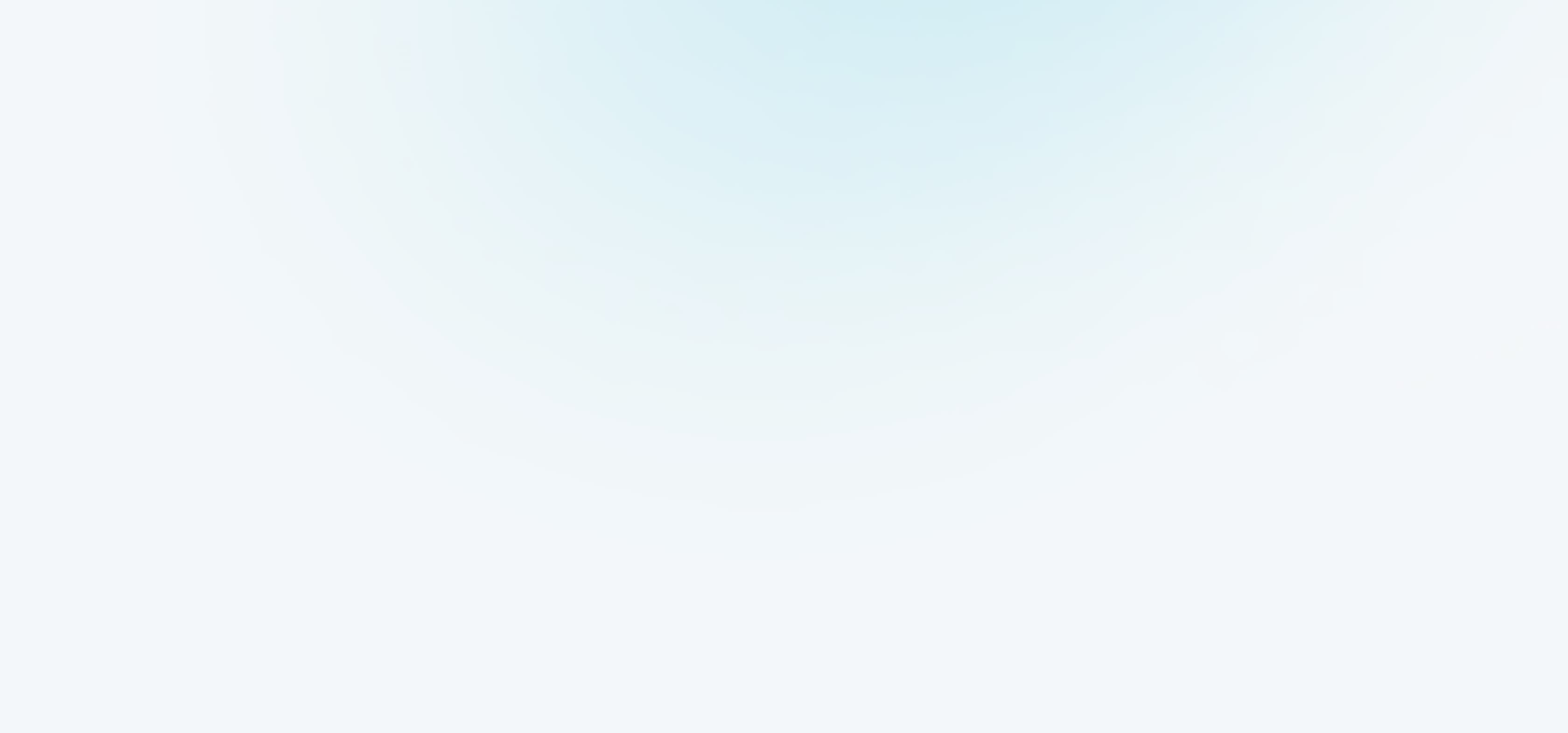
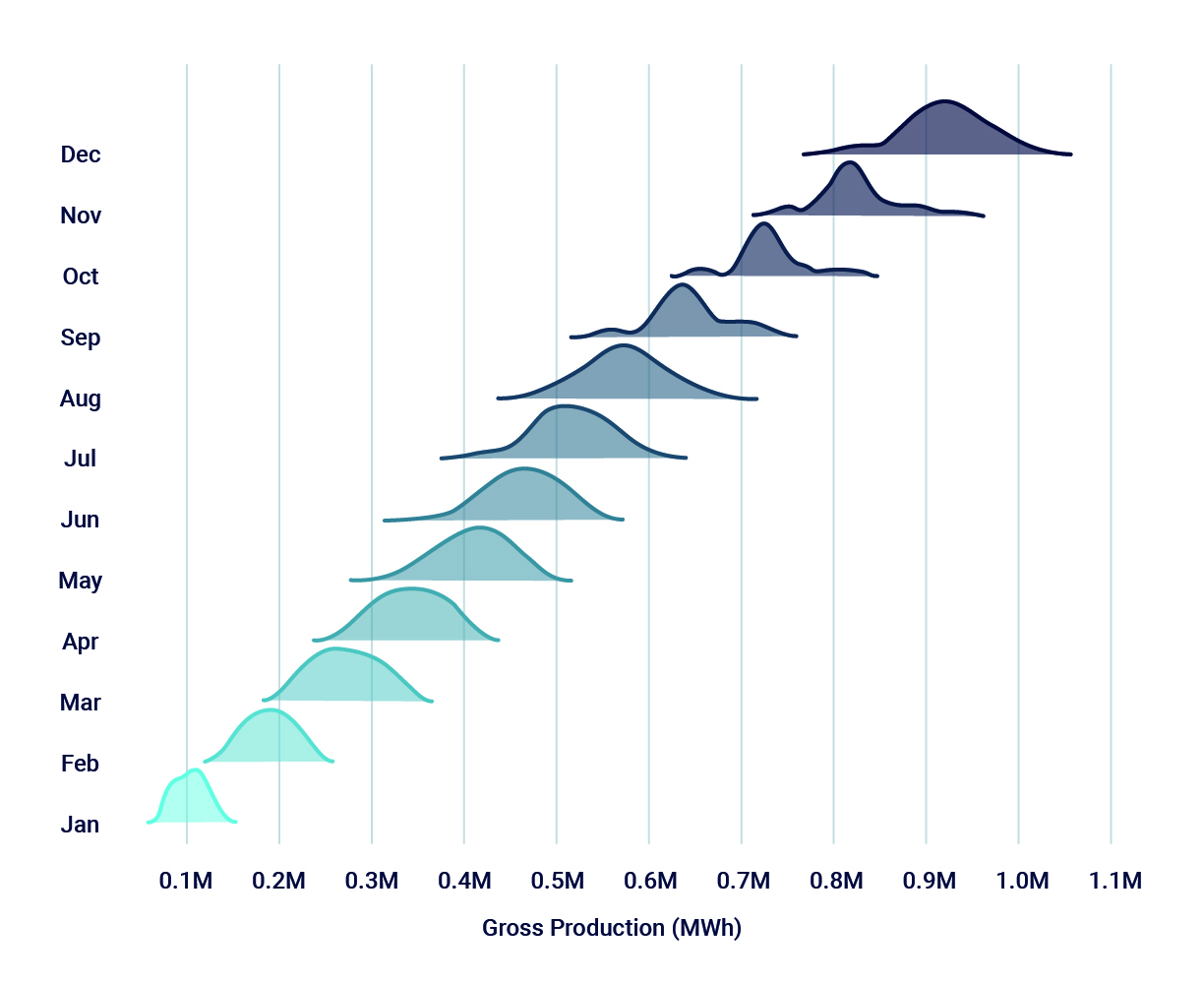
How it works
A machine learning model is trained using hourly gross energy from the farm and climate data from the corresponding period. The projections from the trained model are then tested against actual production in the years following the training data period.
Once trained, the model is used to produce a time series distribution based on more than twenty years of data. The result is a simulation of hourly gross energy production uncertainty bands in any month, quarter or year based on the historical trend.
To provide a more realistic view of hourly production, the model has been further developed to account for the expected energy loss profile of the farm. The validated gross model is trained on industry loss data for equivalent technology of comparable age.
The result is a model of future production taking into account both historical energy and risk to future production based on asset age.
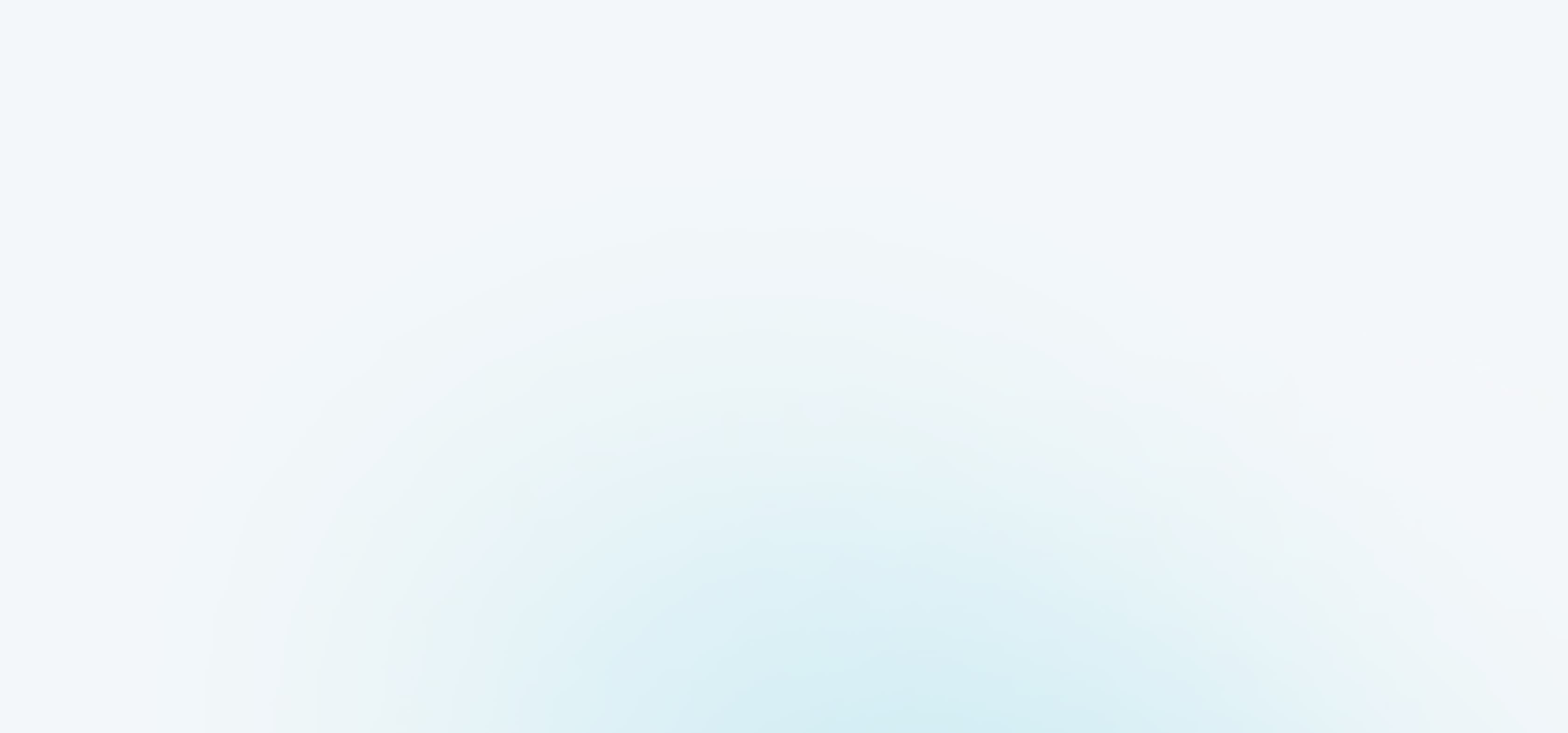